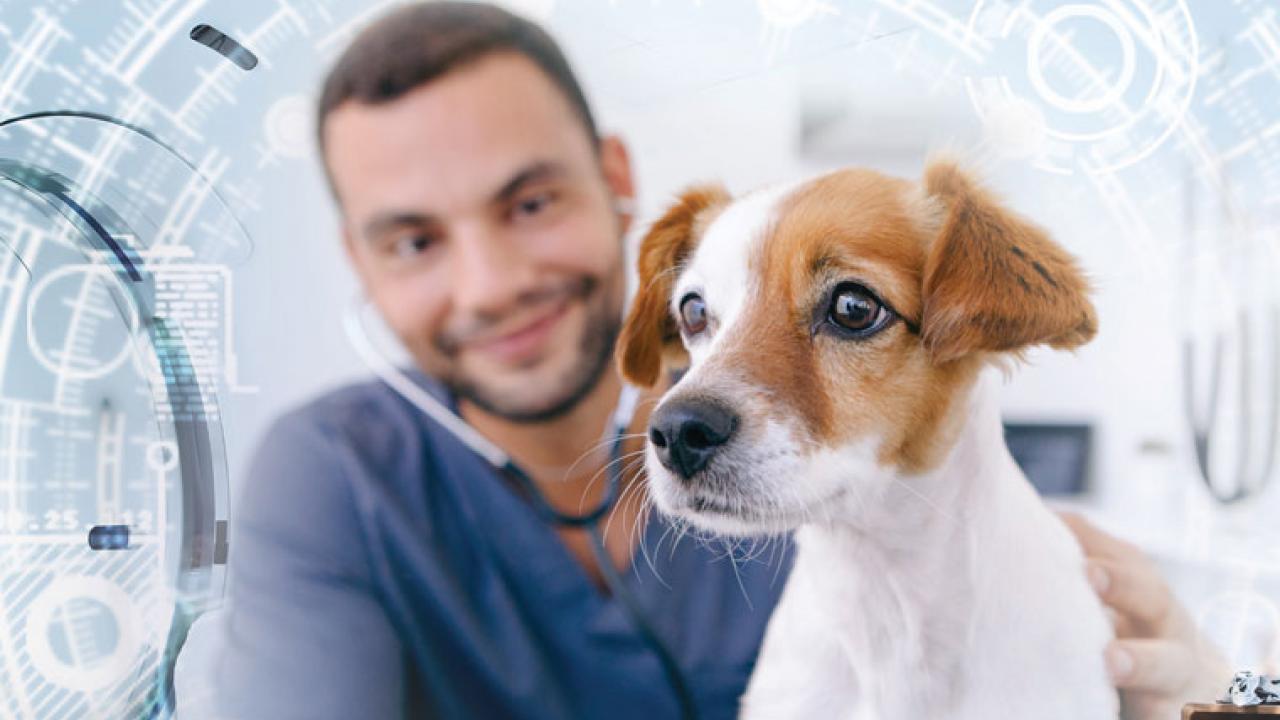
Artificial intelligence & veterinary medicine: Can machine learning live up to expectations?
Written by R. Scott Nolen
Published by the American Veterinary Medical Association
More than a century ago, the automobile ushered in a new age that fundamentally remade the practice of veterinary medicine. Will artificial intelligence do the same?
Virtually every area of life is somehow touched by AI, enhancing our understanding of complex issues and making better outcomes more likely. In the human health care industry, AI is being used in image interpretation, disease diagnosis, patient monitoring, drug development, and even robotic surgery. Google and IBM have invested heavily in the AI health care sector, which is projected to hit $150 billion over the next decade.
Veterinary medicine and pet owners are also using AI technologies, most notably in the areas of radiography, triage, and diagnosis.
Science fact versus fiction
Artificial intelligence is a field of computer science dealing with the simulation of human intelligence through the use of computers.
AI technology rapidly analyzes massive amounts of data according to a set of instructions known as algorithms to accomplish a specific task. These tasks run the gamut, from making an online book or movie recommendation to identifying a person based on their facial features.
And yet, the technology is nowhere near replicating human cognition or creativity. AI can only do what it’s instructed to do, meaning that the algorithms used to sniff out tax fraud can’t also forecast tomorrow’s weather.
Thomas Strohmer, PhD, is a mathematics professor and director of the Center for Data Science and Artificial Intelligence Research at the University of California-Davis. The center promotes interdisciplinary collaborations that use data science and AI to find solutions to some of the world’s most pressing problems, such as climate change and affordable health care for everyone. Dr. Strohmer says there are two types of AI.
“One is a general, very high view of AI that can think and read like humans. This is a grand vision of AI that doesn’t exist yet, right? It’s science fiction and doesn’t exist, not even close,” he explained. “Then you have the more narrow AI that you use for driving cars, language translation, and other really impressive applications. This is what we usually mean when we talk about AI: task-specific AI.
“But I should make it very clear, there is no ‘I’ in AI, not yet. The people who write these algorithms are very intelligent, but the algorithms themselves are not intelligent in a way that they can reason.”
In a 2019 report, the National Academy of Medicine wrote, “AI has the potential to revolutionize health care” and “offers unprecedented opportunities to improve patient and clinical team outcomes, reduce costs, and impact population health.”
The academy also sought to temper expectations about what AI could achieve, however. “One of the greatest near-term risks in the current development of AI tools in medicine is not that it will cause serious unintended harm, but that it simply cannot meet the incredible expectations stoked by excessive hype. Indeed, so-called AI technologies such as deep learning and machine learning are riding atop the utmost peak of inflated expectations for emerging technologies.”
Data analysis
Dr. Krystle Reagan makes it clear that she isn’t a computer scientist. Rather, she’s a veterinary internist at the UC-Davis Veterinary Medical Teaching Hospital and an advocate of AI. She helped develop an algorithm to detect Addison disease with an accuracy rate greater than 99%.
“We call Addison’s disease ‘the great pretender’ because dogs come in with very vague clinical signs. The blood work can look like intestinal disease, it can look like kidney disease, it can look like liver disease. So it’s one of those conditions that you really have to be on your toes,” Dr. Reagan said.
Blood work results from more than 1,000 dogs previously treated at the teaching hospital were used to train an AI program to detect complex patterns suggestive of the disease. The computer program was then able to use these patterns to determine whether a new patient has Addison disease. Dr. Reagan and her team published their findings in the July 2020 issue of the journal Domestic Animal Endocrinology.
Now Dr. Reagan is coding data from canine patients seen at the UC-Davis teaching hospital over the past decade in which leptospirosis was diagnosed or suspected but later ruled out. The project is a collaboration among Drs. Reagan and Strohmer and the center for data science and AI.
“Then we’re using machine learning algorithms to try to identify subtle patterns in the blood work of these dogs that might help us categorize them as having leptospirosis or not earlier than we can with traditional diagnostics,” Dr. Reagan explained.
Timing is important when diagnosing leptospirosis, Dr Reagan said, because the disease can cause serious kidney problems that can become so severe as to require dialysis. “Unfortunately, the gold standard testing for leptospirosis requires two antibody tests about 10 days apart,” she continued.
“The gold standard tells us that I can’t make a diagnosis until at least 10 days after illness. And we really need some sort of tool to help us give owners some guidance in terms of prognosis when we’re looking at this ill dog and deciding whether or not to move forward with dialysis.
“We’re hopeful that we can find patterns in the data that will help us classify our canine patients as having leptospirosis or not—or at least to help us say we think there is an 80% chance that your dog has leptospirosis or we think it’s very unlikely that your dog has leptospirosis.”