The quantity and scope of health data generated is dramatically growing in societal importance and demand for it will continue to rise. Collaborative new research is required, in both the access and application methods, that allows for computing upon these data sets in context, and in secure and responsive ways.
Future use of these data will require new technical architectures that support the legal requirements and the range of evolving users. CeDAR participates in the field of health data research through a focus on potential applications as well as privacy-protecting machine-learning and synthetic data generation and portability.
Using Data Science to Address the COVID-19 Pandemic
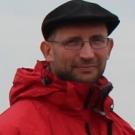
Principal Investigator: Francisco Arsuaga, professor, Molecular and Cellular Biology, Mathematics
Since early 2020, scientists around the world have been working to understand the COVID-19 pandemic, and to develop vaccines and effective antiviral drugs to combat the disease. Viruses like the novel coronavirus behind the pandemic expand their host range via mutation and recombination, becoming more infective and virulent over time, and may acquire a resistance to vaccines.
Arsuaga and his team are developing algorithms to infer the evolution of specific 2019-nCoV genes and their corresponding proteins, with an emphasis on the S-region of the genome. They hypothesize that by using a combination of data science and biophysical methods, we can predict the sequences of the S protein that are most likely to expand the host range of the virus and to increase its virulence through natural selection.
Fine-Grained Uncertainty Quantification of Predictive Algorithms for Healthcare Systems
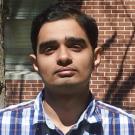
Principal Investigator: Krishnakumar Balasubramanian, assistant professor, Statistics
The ability to predict problems in high-risk healthcare situations can improve the effectiveness and efficiency of the healthcare system, and save lives. Machine learning techniques are starting to be widely deployed in healthcare applications but due to the mission-critical nature of high-risk healthcare applications, predictive algorithms not only need to provide good predictions but also fine-grained margins of error for those predictions.
Balasubramanian and his team are developing predictive machine learning algorithms with fine-grained confidence intervals for imbalanced classification problems arising in healthcare applications. The developed methodology will be applied to two specific healthcare problems: Venous Thromboembolism Episodes (VTE) prediction and postoperative Surgical Site Infections (SSI) prediction.
Machine Learning Assisted Sampling and Patient Classification to Eliminate Paralyzing Bottlenecks in a High-Throughput Raman Spectroscopy Platform for Cancer Liquid Biopsy
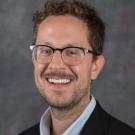
Principal Investigator: Randy Carney, assistant professor, Biomedical Engineering
The classification of circulating biomarkers can improve the ability to detect early-stage cancers like ovarian cancer. Clinical adoption of the techniques and platforms that could perform this task however, is greatly limited by the expense and expertise required to understand and process the incredible wealth of data afforded by these approaches.
Carney and his team have identified key bottlenecks along this pipeline which clever, yet simple, machine learning approaches can be applied to overcome. If successful, the approach in development will enable tracking of tumor growth and resistance to cancer drugs, detect the presence of residual disease and extent of metastasis, and alert physicians to tumor recurrence. Ultimately, advancements made in these areas will transform cancer into a manageable disease.
Needle in Haystack: Fetal Signal Isolation for Transabdominal Fetal Pulse Oximetry
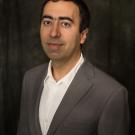
Principal Investigator: Soheil Ghiasi, professor, Electrical and Computer Engineering
The high rate of Cesarean section surgeries in the US, and the associated cost and complications, relative to globally accepted norms is partly due to the unclear picture of fetal hypoxic distress during labor and delivery. UC Davis researchers have been working to build and validate a non-invasive device prototype to enable continuous and convenient transabdominal monitoring of fetal blood oxygen saturation (TFO) during active labor and delivery.
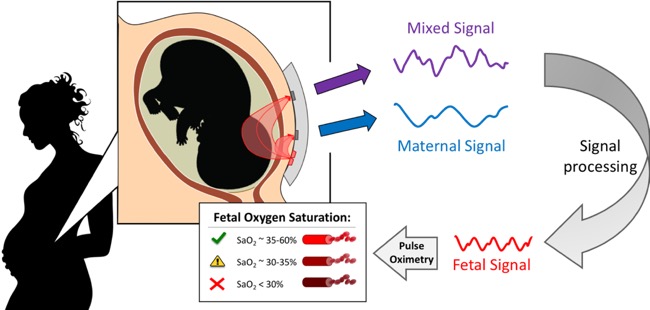
Ghiasi and his collaborators are working to develop and implement superior signal processing algorithms for isolation of weak fetal signal from raw measurements. Fetal signal isolation is an essential precursor to computing fetal oxygen saturation, and its performance is critically important for accurate estimation of fetal blood oxygen saturation.
Artificial Intelligence in Surgical Oncology
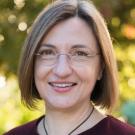
Principal Investigator: Laura Marcu, professor, Biomedical Engineering
Surgeons have to make difficult decisions during a cancer surgery to balance between the removal of cancerous tissue and the quality of life of patients after surgery. By combining rich information from clinical data in the patient’s medical record, pre-operative images, and intra-operative images, AI-based approaches hold great promise to provide intra-operative guidance to surgeons to customize their approach to tissue resection based on patient-specific features.
Marcu and her team are leveraging experimental data from ongoing clinical research studies at UC Davis, and their rich expertise in both head-and neck and brain imaging, to develop an AI model to augment surgical decision making by combining patient history, standard pre-operative imaging and a novel intra-operative optical imaging technique; and to develop an AI model for identifying factors associated with good surgical outcome.
Democratizing Health Research Through Privacy-Protecting Synthetic Data
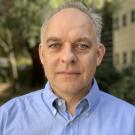
Principal Investigator: Thomas Strohmer, professor, Mathematics
There is intense demand from academic, industry and government organizations to access and use large scale health data, however gaining access to high-quality health data of sufficient size, representative heterogeneity, and with sufficient descriptive metadata remains a laborious and broadly frustrating process. Synthetic data is a promising approach that can lead to the democratization of access to and use of clinical and health data beyond its current legal and ethical constraints.
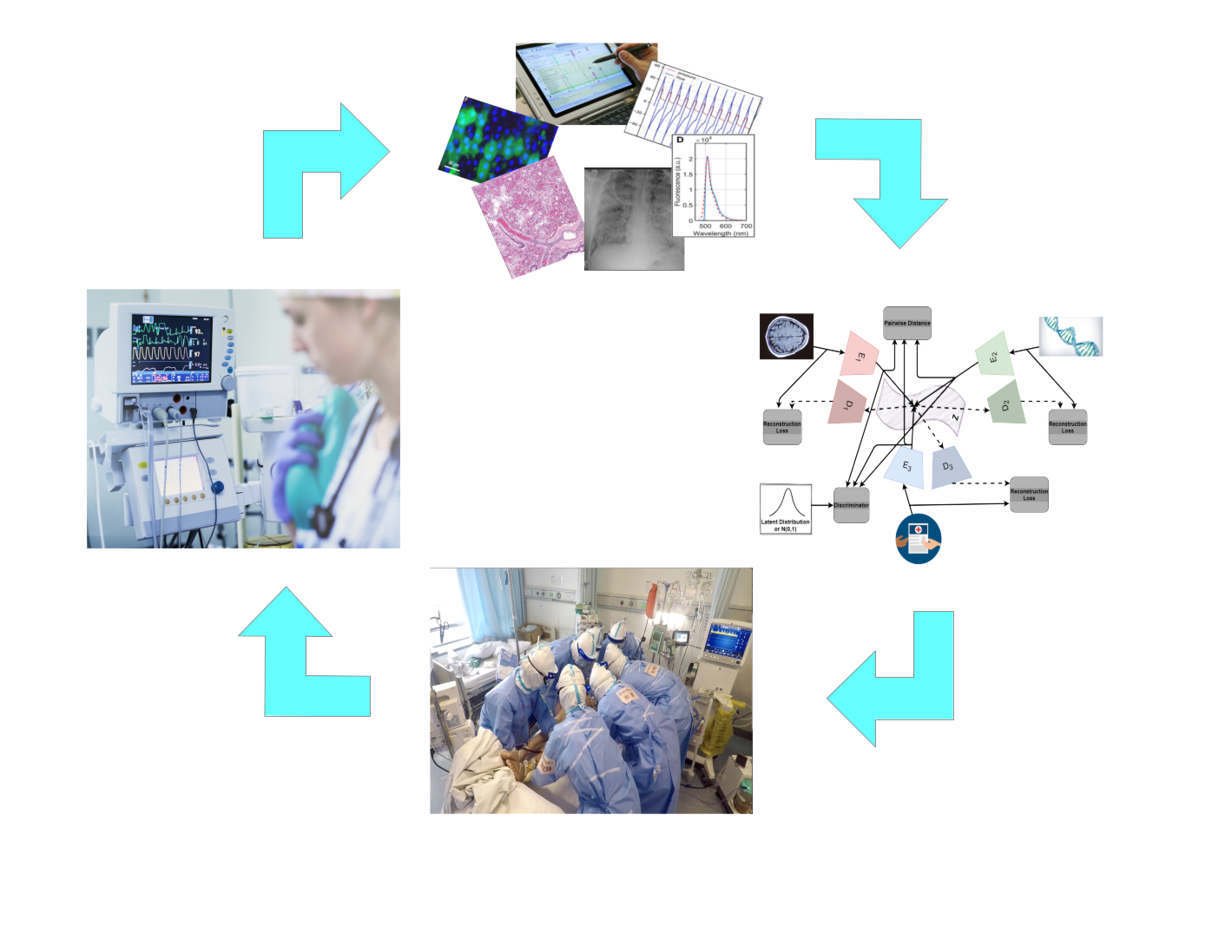
Strohmer and his team are developing a rigorous mathematical framework for the faithful and privacy-preserving generation of synthetic multimodal health data. This framework will help advance health research using tools from AI and machine learning while at the same time ensuring that fundamental rights of privacy are upheld.