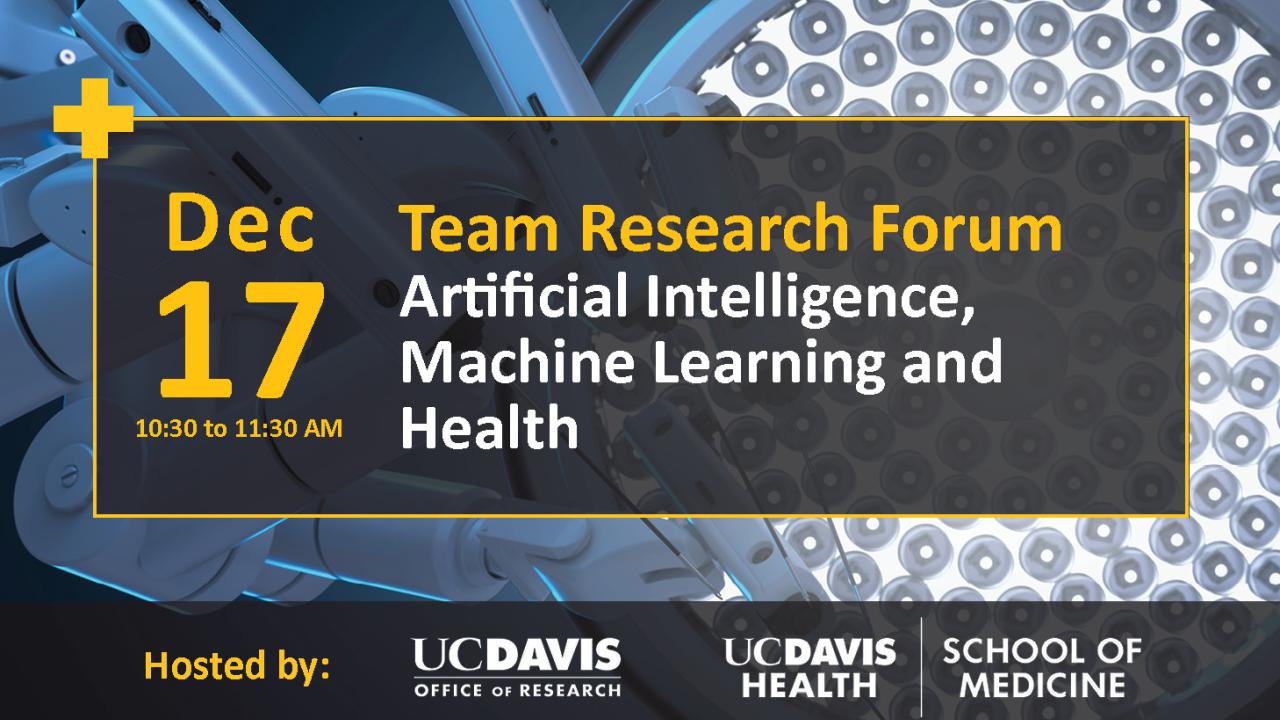
Event Date
Join the UC Davis Office of Research and the UC Davis School of Medicine in a discussion about artificial intelligence and machine learning research applications in health at UC Davis. UC Davis researchers will present research they are currently working on in this field following an overview of funding opportunities and resources for research teams working on artificial intelligence and machine learning research with health applications.
Experimental Data Science in Smart Health Domain: Lessons Learned & Opportunities
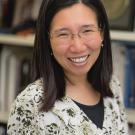
Dr. Chen-Nee Chuah
Child Family Professor in engineering
Department of Electrical and Computer Engineering
UC Davis
Data science and statistical/machine learning techniques can be leveraged to tackle various predictive and decision control problems in a wide range of application domains. This talk will focus on opportunities and challenges that arise in applying IoTs, big data, AI, and machine learning (ML) techniques to smart health domain such as AI-assisted predictive analytics and critical patient care, medical imaging, and video-based behavior detection. Specifically, we will draw examples from our on-going collaborative projects with UC Davis Medical Center, the Alzheimer Disease Center, and the MIND Institute. We will walk through end-to-end data analytic pipeline that involves automated processing of physiological signals, web interface for expert annotations, rule-based feature extraction, and the development of ML and deep learning models for disease detection. We have had promising results applying this pipeline to (i) detect acute respiratory distress syndrome (ARDS) in patients under mechanical ventilation, (ii) predict fluid responsiveness in hemodynamically unstable patients, and (iii) screen for rare critical congenital heart disease in newborns.
Democratizing Health Research for Critical Care
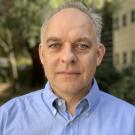
Dr. Thomas Strohmer
Director, Center for Data Science and Artificial Intelligence Research (CeDAR)
Professor
Department of Mathematics
UC Davis
The intensive care unit, and by extension the whole area of critical care medicine, is becoming one of the most data-driven clinical environments. However, due to privacy constraints, lack of availability and access to sufficient data is thus quickly becoming a main road block for medical experts and machine learning scientist towards the development of sophisticated medical decision support systems. Synthetic data is a promising concept for solving this conundrum, by reconciling data innovation with data privacy, while avoiding the pitfalls of standard anonymization techniques. The aim of this project is the development of an algorithmic framework for the faithful and privacy-preserving generation of heterogeneous, multimodal synthetic datasets to boost the development of clinical decision support applications. Special emphasis is placed on usage and validation of our approach for datasets arising in critical care, using the Acute Respiratory Distress Syndrome as a model of conditions requiring multimodal data for diagnosis. This is a joint project with Rachael Callcut (Division Chief, Trauma, Acute Care Surgery and Surgical Critical Care) and Jason Adams (Department of Internal Medicine, Division of Pulmonary, Critical Care, and Sleep Medicine) at the UCD School of Medicine.
AI-based Sensing for Healthcare Applications
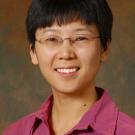
Dr. Xin Liu
Professor
Department of Computer Science
UC Davis
In healthcare applications, medical devices, or sensors, have been widely used to measure physical, biological, neurological, and chemical characteristics of patients. Such sensors include accelerometers, cameras, walking plates, EEG electrods, ultrasound, CGM, etc. AI-based analytical tools can contribute to the analysis of such time-series data collected by these various sensors. This enables medical practitioners, as well as patients themselves, to better understand the physiological performance of patients. In this talk, we focus on AI-based sensing for healthcare, using examples from our on-going collaborations, including 1) an iPhone-based system for DMD (Duchenne Muscular Dystrophy) identification, currently in clinical use, based on accelerometer data; 2) motor dysfunction quantification based on force plate and camera data; 3) ADHD patient analysis using actigraph, 4) Sonomyography based on ultrasound, and 5) brain activity classification based on EEG.
DataLab's Health Data Science Research Collaborations
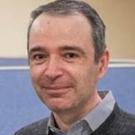
Dr. Vladimir Filkov
Professor
Computer Science Department
Director for Translational Data Science, DataLab
UC Davis
Over the past several years DataLab has made concerted efforts to grow its health data science research and training activities in order to address growing demand by UCDH researchers. In this talk I will describe the different types of medical research needs we encounter, outline our multi-pronged approach to health research collaborations, and give examples of our ongoing projects with UCDH research and operations teams. I will finish with challenges and opportunities in growing a health data science research service.